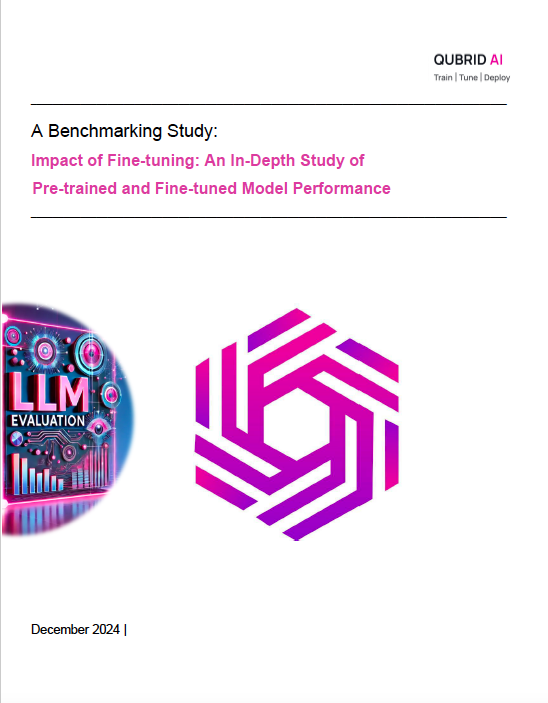
Abstract
In this white paper, we present an in-depth evaluation of fine-tuned large language models (LLMs) from the Llama series, specifically focusing on Llama 3.1 8B Instruct, Llama 3.1 70B Instruct, Llama 3.2 1B Instruct, and Llama 3.2-3B Instruct models. These models were fine-tuned using Qubrid AI’s proprietary question-answer dataset, designed specifically for training in question-answering tasks. Throughout this study, we analyzed the models’ performance through comprehensive evaluations, including ROUGE metrics to assess summarization quality and compare each fine-tuned model’s performance against its base model. Performance insights are provided via training loss graphs, as well as a comparative analysis of ROUGE scores across models. Our findings offer valuable insights into the efficacy of fine-tuning approaches for Llama models in question-answering tasks, informing future optimization efforts and applications in similar NLP tasks. Download the pdf white paper below: